While these measures will be highly effective in terms of protecting lenders and their customers, the predictive and forward-looking nature of IFRS 9 required a significant evolution of previous provisioning approaches.
The question for lenders is how to calculate ECL under IFRS 9, how to effectively predict and report significant changes to credit risk, and how to minimise the significant time and cost associated with building and running IFRS 9-compliant ECL models.
What is IFRS 9 and what does IFRS 9 mean for banks and other lenders?
IFRS 9, which came into force in 2018, is a set of financial forecasting and reporting accountancy standards that specifically address how credit risk losses should be reported in accounts. Unlike many other financial services regulations, IFRS 9 applies to all listed organisations with lending products, from banks and building societies, to credit card providers, retailers, and payday loans companies.
There’s a significant difference between IFRS 9 and IAS 39. This is because IFRS 9 is predictive and forward-looking, requiring reporting of SICR before customers miss a payment. As a result of this critical difference, IFRS 9 ensures that companies recognise their potential future losses more accurately in their accounts and cannot delay the recognition of asset impairments which was a criticism of IAS 39 during the financial crisis where accounts could not recognise losses until there had been a missed payment.
To ensure that ECL forecasts are calculated as accurately as possible, IFRS 9 financial instruments require ongoing calculations of Probability of Default (PD), Exposure at Default (EAD), and Loss Given Default (LGD). IFRS 9 also defines three different “Stages”, performing, underperforming and non-performing. ‘Up to date’ customers who haven’t shown a significant increase in risk since opening are Stage 1 or performing accounts, those who have shown a significant increase in risk fall into Stage 2 or underperforming, alongside customers who are 30 days or more past due. A customer who has defaulted, based on a rebuttable presumption of being 90 days past due, is allocated to Stage 3 or non-performing. The main difference between the different stages is that a 12-month definition of PD is used for accounts at Stage 1, a lifetime definition of PD is used for accounts at Stage 2 and the PD is 100% for Stage 3 accounts.
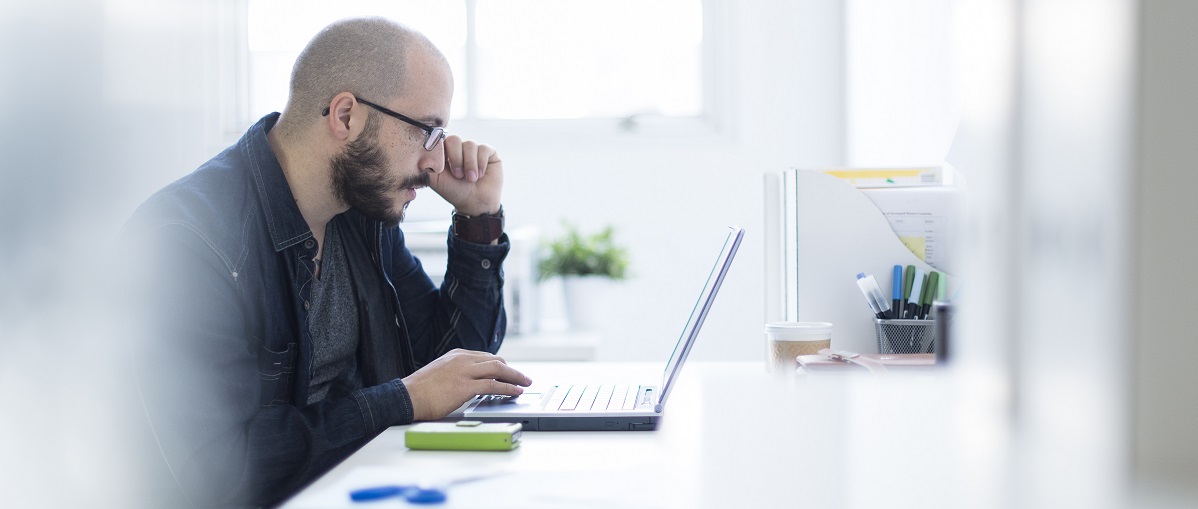
With a view to supporting accurate, forward-looking ECLs, IFRS 9 has resulted in a number of key modelling and forecasting challenges. Among the most important of these are:
- Use of historical default data for ECL forecasts
Lenders need to have access to a sufficient volume of historical default data to build robust PD models. This does not just have to be collected in house but can be augmented by, or be sourced from, data for similar lending products and portfolio demographics.
- The ability to calculate ECLs based on multiple credible economic scenarios
ECLs must reflect a supportable view of forecast macro-economic conditions and the impact that those economic conditions will have on the future portfolio losses. To comply with IFRS 9 auditing requirements, economic scenarios must be credible and probability weighted.
- Auditor’s requesting ‘sensitivity’/’materiality’ testing
Lenders are being asked to modify and rerun models to establish the materiality of the underlying assumptions that feed into the ECL model – recalculating ECL under different assumptions to understand the impact on ECL. Likewise, adjustments and model reruns are often requested by IFRS 9 auditors to assess the sensitivity of models to changing credit risk factors.
- Model validation based on peer ‘benchmarking’
Auditors are asking lenders to reference ECLs from peer organisations to “justify” their own approaches and calculations. This is incredibly difficult to do from published annual reports. IFRS 9 is an example of principle based regulation meaning that almost all the elements of the calculations are open to individual lender interpretation that, coupled with the ability to incorporate manual overlays, means that comparing annual report numbers is a case of comparing “apples and giraffes”.
- The ability to detect SICR across the portfolio
Calculated ECLs are expected to be forward looking, and reflective of expected risk. Indicators of changing risk, for example missed payments, should be considered to ensure appropriate calculations. Whilst this can sometimes be done from internal account level behaviours it often requires lenders to look beyond their specific portfolios to understand when customers are becoming financially stressed, either by consuming data from a credit bureau, or other data sources that indicate the customers’ current financial situation and ability to repay.
The forward-looking nature of IFRS 9 has led to increased focus on ECL forecasting across all kinds of lenders, with new levels of collaboration between finance and risk teams. However, many lenders have also found it costly and time consuming to build ECL models that can be easily modified and flexed to meet the new, predictive forecasting requirements of IFRS 9.
In the following sections of this guide, we look at the key challenges’ lenders face in their IFRS 9 journeys and outline several solutions and approaches that can reduce the workloads and costs often associated with ECL modelling and forecasting.
Top IFRS 9 implementation challenges
Although IFRS 9 is mandatory because it is principles based regulation many lenders faced significant challenges in terms of adapting to the new rules and achieving compliance. While some banks have been able to adapt their Basel capital models to achieve IFRS 9 compliance, many lenders have been faced with the prospect of building bespoke IFRS 9 ECL models from scratch, which required significant resource, time and cost.
Key challenges for lenders on their IFRS 9 compliance journeys include:
- A lack of relevant historic and/or default data for ECL modelling
Collecting and analysing the right data to support IFRS 9 compliant ECLs is a significant challenge for lenders. A long time period of historic data is required to build a picture of the probability of default and link it effectively to the macro-economic factors that impact credit losses over time. Having sufficient historic data can be a real challenge, especially if lenders are new to the market or launching a new lending product. For others, such as mortgage providers, the extremely low level of defaults in recent years means that data is lacking for any meaningful future ECL analysis, forecasting and robust model development. Similarly, some lenders have closed books for which available data is limited or becoming more limited.
Lenders can also experience difficulties if their data providers are unable to segment customer risk profiles effectively based on their demographics, including their age and where they live. With the Covid-19 pandemic particularly impacting certain age groups and regions, this kind of segmented data provides extremely valuable insights that are pertinent to economic forecasting.
- Models built on high level macro-economic forecasts
As well as experiencing a data shortfall for IFRS 9-compliant ECL modelling, many lenders also lack the credible, probability weighted economic forecasts needed to effectively predict credit losses across their portfolio. While economic forecasts can be procured from economics houses these often lack the links to the underlying credit losses that are required for best practise IFRS 9 compliance.
In addition, the economic scenario probability weightings used are often subjective rather than based on quantitative analysis. These can be difficult to justify during audits.
- Lack of in-house resources and skills, resulting in high ECL modelling costs
Many lenders can lack the in-house resources and skills needed to build and manage IFRS 9-compliant ECL models. This often results in lenders engaging third-party consultancy firms at an extremely high cost to the business. For mortgage lenders and others with a low rate of default, the cost of hiring consultants to build bespoke ECL models can even outweigh total losses from customer defaults, representing a lost investment opportunity for the business.
The COVID-19 pandemic has brought common IFRS 9 challenges into sharp focus
The COVID-19 pandemic caused major unprecedented disruptions to normal economic activities around the world. However, the effects of these economic disruptions are not consistent with previous experience in terms of their impact on credit risk losses. This is due to the temporary nature of the disruption, coupled with government interventions, such as the furlough scheme and payment holidays. For example, if lenders used GDP as a macroeconomic variable in their ECL models they are experiencing unexpected volatility in their forecasts as GDP plunged -20% in the second quarter of 2020 only to rebound by +12% in the third quarter whilst previously observed maximum falls in GDP were in the region of only -2%. Disruptions caused by the pandemic are also disproportionately impacting some age groups, occupations and regions more than others, significantly undermining some of the blanket assumptions about the potential economic impacts on credit losses used in some ECL calculations.
The sudden and complex nature of the pandemic, and its varying impacts on different socio-economic groups, mean that many lenders are finding it difficult to adjust their ECLs in a timely, accurate, and auditable way. The challenge is also being exacerbated by inflexible and complex bespoke ECL models that are time consuming to change, run and maintain.
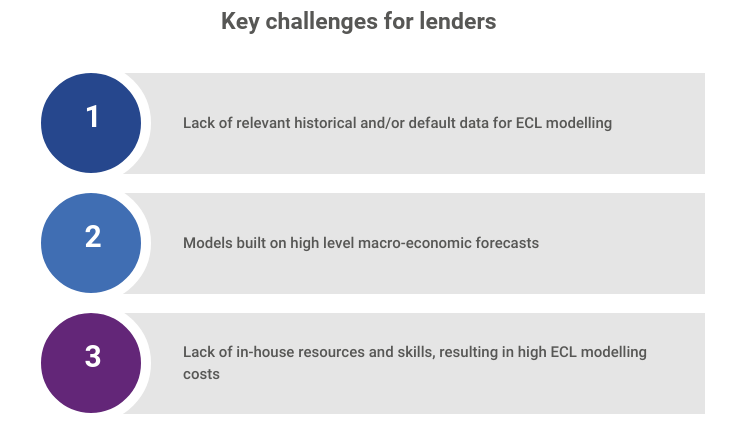
Key considerations for a successful IFRS 9 compliance strategy
To address the challenges of IFRS 9 compliance, especially in the current turbulent economic climate, organisations need to ensure that their ECL models are flexible, efficient to build and run, and – critically – based on sound and justifiable underlying assumptions and macro-economic forecasts.
To ensure that ECLs continue to protect the organisation and its customers, while also satisfying the requirements of IFRS 9 auditors, organisations need to:
- Integrate data sources that can proactively detect SICR
To better meet the requirements of IFRS 9, lenders can integrate multiple data sources, such as credit bureau data and affordability data. This kind of data can show, for example, if a customer is reaching their overdraft limit each month, if they’re spending heavily on credit cards, or if they’re searching for additional credit options and products – all signs that they’re becoming financially stressed. This kind of insight ensures that SICR is accurate for a lenders specific portfolio based on their customer profile.
- Enhance ‘peer benchmarking’ for ECL models
Currently, benchmarking is conducted by reviewing peers’ and competitors’ annual reports manually. As other organisations may use different measures and assumptions to calculate ECLs, and have different customer demographics, this kind of analysis rarely achieves a ‘like for like’ comparison and can lead to misleading conclusions. Lenders can resolve this challenge by reviewing a comparison portfolio using the same ECL modelling factors including the same probability weighted economic forecasts and including credit bureau data – which can be used to explain why ECLs differ from peer results.
- Architect ECL models that can flex and adapt
By building flexibility into ECL models, lenders can alter underlying assumptions more quickly and efficiently with no need to rebuild models from the ground up. This approach also reduces the need to override ECLs using ‘management overlays’ which can be difficult to justify to the auditors.
- Integrate data that shows the differing impact of macro-economic changes on specific customers or customer segments
Integrating credit bureau data, especially demographic and current account turnover (CATO) data, into ECLs, can help lenders better understand how macro-economic events are impacting different types of customers. In the case of the Covid-19 pandemic, this kind of data shows that younger people and people in certain regions have been most affected financially, while older people have typically been less affected. As a result of the pandemic it’s becoming increasingly important for lenders to factor in the impact of new information such as emergency payment holidays, income shock and increasing unemployment risk in ECLs.
- Use credible, probability weighted macro-economic forecasts
This is essential for accurate ECL calculation and for compliance with IFRS 9 requirements for projections that are truly forward-looking and go beyond ‘everything staying the same’. It really helps if the economic scenarios have narratives attached to them to provide justification to the auditor, and if the ‘probability weighting’ is based on a quantifiable likelihood of a specific scenario coming to pass. This will remain critical as the on-going impact of Brexit needs to be factored into economic outlooks in addition to the pandemic effects.
- Simplify ECL modelling where possible to reduce workloads and costs
In some cases, lenders need complex, bespoke models to accurately forecast and report ECL. However, this is not always the case, and many smaller lenders, or lenders in low-risk markets, could potentially use far simpler models and tools to build ECLs in a fully IFRS 9-compliant way, saving costs and contributing directly to the bottom line.
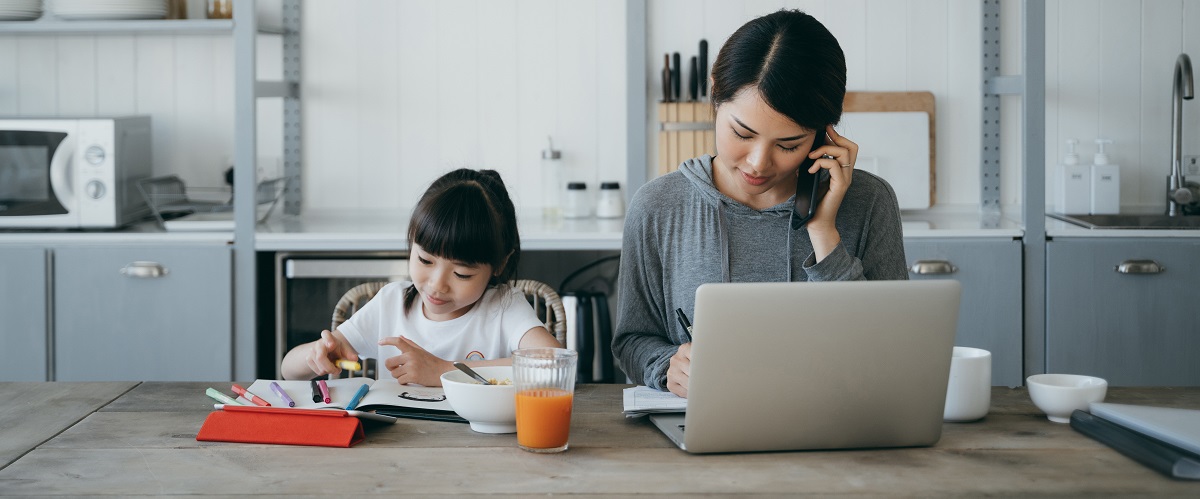
How Experian can help lenders accelerate their IFRS 9 compliance journeys
Experian can help lenders to enhance their ECL models and streamline IFRS 9 compliance with a unique combination of data insights, technology tools and professional services. With a broad portfolio of products and capabilities, we can address the needs of all lenders, whether they’re experiencing a range of IFRS 9 compliance challenges, or if they need specific help with one area of their ECL modelling.
Key Experian capabilities and offerings that can help lenders enhance the accuracy of their ECLs, reduce ECL modelling workloads and costs, and streamline IFRS 9 compliance, include:
- The Experian Credit Loss Insight on-demand software tool
Our on-demand IFRS 9 Credit Loss Insight (ICLI) tool enables lenders to calculate IFRS 9 Expected Credit Losses for their portfolio in just a few clicks. ECLs are based on CAIS data and Delphi customer level risk scores, combined with industry-leading, forward looking, and probability weighted economic scenarios.
With Experian ICLI, lenders can:
- Calculate IFRS 9 components on their own portfolios based on robust CAIS samples
- Automatically incorporate regularly refreshed, probability weighted economic scenarios into ECL calculations
- Streamline their IFRS 9 audit processes
- Reduce reporting workloads and costs
- Save time and resources vs. building and implementing bespoke ECL models
Watch the demo below where Experian’s Liz Clarke, Head of Regulatory Analytics, and Alastair Porter, Senior Analytics Consultant, show how this new tool works and how it can help you.
ICLI includes Experian’s probability weighted economic forecasts
Economic forecasts from Experian’s team of expert economists provide a solid and auditable foundation for ECL models and forecasts. Each suite of economic forecasts includes a ‘baseline’, ‘upside’ and ‘downside’ scenario and is delivered with a full narrative explaining the underlying economic assumptions, allowing lenders to easily explain model outcomes to their auditors.
Each suite also includes stochastically derived probability weightings that accurately reflect the range of outcomes that might be expected given the current macro-economic information available.
Experian is an award-winning economics forecasting house as rated by Consensus Economics.
- Data provision
We can provide insights such as consumer delinquency rates, segmented company default rates, total market default values, and more, helping lenders to overcome challenges of insufficient historical default data.
- Tailored IFRS 9 sample profiling
We can provide generic, anonymised data to be used for analysis, or to augment existing internal data to increase robustness of results. Allows lenders to build more accurate ECLs by ensuring that underlying assumptions match their customer profile and product types as closely as possible (across product types, loan terms and amounts, and prime vs. sub-prime products).
- Bespoke ECL model development support
Our bespoke support allows lenders to build their own ECL models more quickly and efficiently, with less need for internal resources. Experian can provide outsourced analytics or bespoke modelling for any or all the IFRS 9 component models from PD, EAD, LGD and stage allocation, including SICR.
- Economic modelling and forecasting services
Lenders can take Experian’s award winning regular economic forecasting services which supplies credible economic scenarios with associated stochastically derived probability weightings and easy to interpret narratives. In addition, Experian can also build models that link the appropriate macro-economic variables to the lenders credit risk variables enabling lenders to model the impact of economic scenarios on their ECLs, replacing the requirement for subjective and hard to justify manual overlays.
- ECL model reviews and evaluations
Experian provides independent model or component review and validation services, and we support audit conversations on behalf of our clients.
- Benchmarking
Experian can provide a benchmarking module to ICLI that enables lenders to model a peer group portfolio using the same ECL modelling factors including the same probability weighted economic forecasts and then compare detailed results. This can be used to help explain why ECL models differ from other lenders.
The forward-looking ECL forecasting requirements of IFRS 9, plus major events such as Covid-19 and Brexit are creating a perfect storm for credit risk and finance teams at lenders of all types and sizes. In many cases, traditional or legacy approaches to credit loss reporting are no longer agile or cost effective enough – requiring significant resources and time to maintain, adapt, and re-run.
To address these and other challenges, many lenders are now opting for more agile solutions, such as Experian’s on-demand Credit Loss Insight tool, which can reduce the cost and time associated with building and maintaining bespoke ECL models. At the same time, IFRS 9 requires a new level of transparency and accountability across all underlying assumptions, including economic forecasts, which can require support from experienced partners who can not only provide these, but also support the audit journey to minimise time and cost risks.
Experian is working with some of the UK’s leading lenders to help reduce the burden of IFRS 9 compliance, and to improve the accuracy of credit loss reporting across a range of product types and portfolios. To find out how we can help you do the same, please contact us.